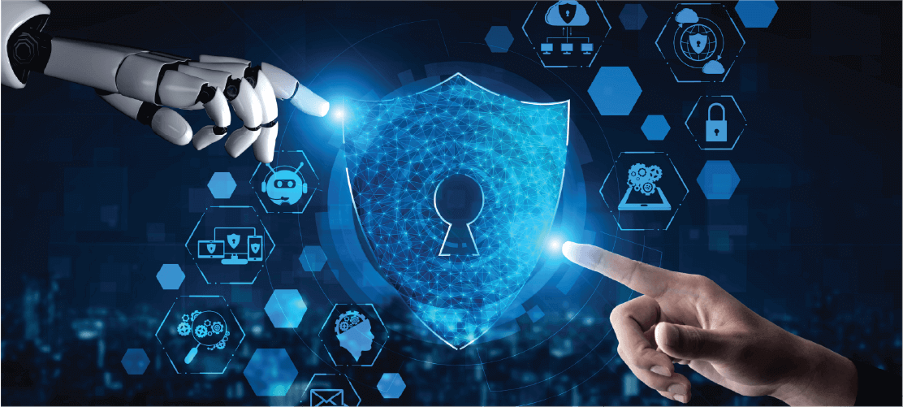
- Proactive Defense: Machine learning models predict potential attack vectors by analyzing historical data and patterns.
- Examples:
- Forecasting phishing campaigns based on email traffic.
- Anticipating malware evolution and updating defenses.
3. Automated Response
- Real-Time Mitigation: AI can automatically contain threats, such as isolating infected devices or blocking suspicious traffic.
- Examples:
- AI-driven firewalls that adapt to new threats.
- Automated removal of malicious code or quarantining compromised files.
4. Behavioral Analysis
- User and Entity Behavior Analytics (UEBA): AI models baseline normal user behavior and flag deviations.
- Examples:
- Detecting insider threats when employees access unusual files.
- Identifying bots or automated attacks mimicking human behavior.
5. Security Orchestration
- Streamlined Workflows: AI integrates multiple cybersecurity tools to improve efficiency.
- Examples:
- Coordinating threat intelligence sharing across platforms.
- Automating incident reporting and prioritization for IT teams.
AI in Cybercrime
While AI is a powerful tool for defense, it also introduces new risks:
1. AI-Powered Cyberattacks
- Sophisticated Phishing: AI-generated messages mimic human behavior to fool victims.
- Deepfakes: AI creates convincing audio or video impersonations for fraud or misinformation.
- Automated Malware: AI enables malware to adapt and evade detection.
2. Vulnerabilities in AI Systems
- Adversarial Attacks: Hackers manipulate AI models by feeding them misleading data to bypass defenses.
- AI Bias Exploitation: Attackers exploit weaknesses in poorly trained AI models.
Advantages of AI in Cybersecurity
- Speed and Efficiency: AI can process and respond to threats faster than humans.
- Scalability: AI handles vast amounts of data, protecting large and complex networks.
- Continuous Learning: Machine learning models improve over time by learning from new threats.
Challenges of AI in Cybersecurity
- False Positives and Negatives: AI systems may incorrectly classify benign activity as malicious or fail to detect threats.
- Cost and Complexity: Implementing and maintaining AI systems can be expensive and resource-intensive.
- Dependence on Data Quality: AI’s effectiveness depends on the quality and quantity of training data.
Use Cases of AI in Cybersecurity
- Intrusion Detection Systems (IDS): AI flags unauthorized access attempts in real time.
- Email Security: AI filters out phishing emails and spam.
- Fraud Prevention: AI detects fraudulent transactions in financial systems.
- Endpoint Security: AI protects devices like laptops and smartphones from malware.
- IoT Security: AI secures connected devices by monitoring unusual activity.
Future Trends
- Explainable AI: Making AI decisions in cybersecurity more transparent and understandable.
- AI-Driven Threat Intelligence: Enhanced collaboration across industries using shared AI insights.
- AI and Blockchain: Combining AI with blockchain for more secure data sharing and identity verification.
AI is transforming cybersecurity by enhancing defenses against increasingly sophisticated cyber threats. However, it requires careful management to maximize benefits while addressing risks and challenges. Let me know if you’d like guidance on tools or implementation!